Dean DeBiase is a best-selling author and Forbes Contributor reporting on how global leaders and CEOs are rebooting everything from growth, innovation, and technology to talent, culture, competitiveness, and governance across industries and societies.
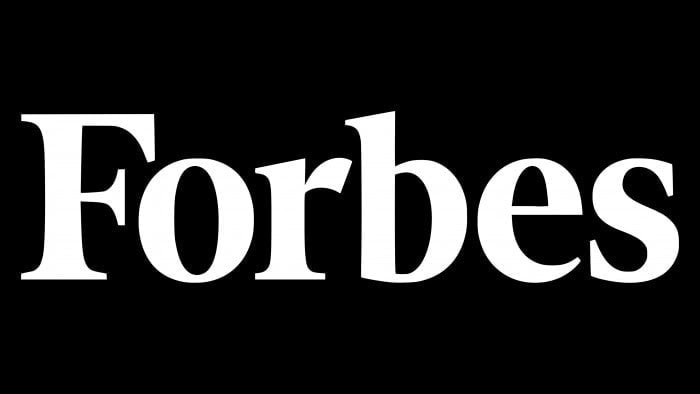
CXO’s Move Beyond Experimentation In GenAI Adoption Game

By Dean DeBiase
July 16th, 2024
The boom of generative AI (GenAI) has prompted businesses across banking, finance, retail, travel, telecom and beyond to explore its potential for gaining a competitive edge. As I’ve previously reported, the technology has been rapidly evolving and improving and now the race is on for corporations to find a fit.
The accelerated pace of optionality can be overwhelming, like a rapid-fire game of Tetris®, as CXO’s look to deploy these increasingly advanced models in AI-based products and services.
Most CXO’s I talk with seem frustrated as they continue to experience challenges on the road to reaping GenAI goodness. It is all too easy to box yourself in with the wrong choices or become one of the many highly visible fails that we have written about at Forbes. Despite the hype around GenAI, a McKinsey & Co. report states that only “11 percent of companies have adopted Gen AI at scale.” Why is this—and what can a CXO do about it?
50 Ways To Leave Your AI Project In The Dust
I often write and speak on the importance of taking a strategic approach when rolling out the latest AI. But what does that mean, exactly? What are the rules of the game? And how can things go wrong?
They’re key questions, as enterprises are struggling to move beyond the initial experimentation stage, with the prototype-to-production lifecycle averaging around eight months, according to Gartner. High costs, concerns over data privacy and security, and technical complexities, including system integration and the need for skilled AI talent, create large barriers to entry.
Those are just some of the challenges for CXOs. While it may not be hard to apply GenAI to very targeted areas or groups, tapping and unleashing its power across the enterprise spanning multiple use cases, with just the right models, integration and fine tuning is no small task.
Just think about it. Here are some of the boxes CXOs need to check off in accomplishing such a feat.
A moving target: Generative AI solutions appear in the market seemingly overnight. Technical teams are challenged to find robust tools that simplify the AI development process from inception to deployment.
Model selection and fine tuning: Configuring perfect prompts, adapting the best models, and testing and ensuring an enterprise-ready solution makes it very time-consuming. But it might not be easy to find and use models that are community, open-source or application-specific. Sourcing and implementing inappropriate models can have a detrimental effect on business outcomes.
Overcoming integration hurdles: Technical complexities and extended development cycles represent significant obstacles in AI integration. These challenges are primarily due to the need for specialized skills in managing sophisticated AI models and the substantial time investment and staffing required to ensure these systems are both effective and reliable.
A fragmented space: Most tools available today are offered as separate solutions, making collaboration difficult. Technical teams must either find something turnkey or cobble together solutions that eventually work together.
Avoiding lock-in: The flip side of this are monolithic solutions from big tech, the hyperscalers like Azure, Amazon, Google et al that might check off a number of boxes but also box you in, limiting choices and opportunities for growth.
From infrastructure to front-end: You need to cover the bases, regarding integration with cloud, backend systems, data, workflows, and user interface. You need an easy way to generate AI agents and tap APIs.
Two critical paths that can throttle projects if not addressed:
1. Progressing from experiment to pilot to production.
2. Scaling a solution enterprise-wide.
The Market Responds To GenAI Adoption Needs
There are a growing number of options to address the obstacles mentioned above.
E.g., cloud environments like HuggingFace, Google Cloud, Azure and others offer extensive cloud and GenAI capabilities. There are also model providers like Cohere, OpenAI and AI21 Labs that have generative AI products which are tied to their models.
The LLM/Generative AI market is saturated with flow builders, while data management is gaining overdue attention. Ops-centric frameworks focus on the efficient, reliable, and secure operation of large language models (LLMs) in production, ensuring optimal performance and scalability.
Then, there are hub, data and flow-centric platforms that support the development and orchestration of LLM and Generative AI applications, emphasize data discovery, design and development, and build flows for conversational use, respectively.
If this all sounds a bit much, remember not all CXO’s need to know how AI works—they just need to learn ways to adopt technology to change the game and transform their business competitiveness.
Find Partners To Help Close Your GenAI Adoption Gap
Back in January, I wrote about the NVIDIA (NVDA)-backed conversational and generative AI company, Kore.ai, that offers AI platforms for enterprises. After its $150 million funding round, the company seems to have jumped in to tackle head-on the GenAI adoption challenges enterprise CXOs are facing.
With its eyes set on surpassing the tech giants and upstart rivals, today Kore.ai announced and launched GALE(Generative AI and LLM Platform for Enterprises), an end-to-end platform enabling enterprises to innovate, experiment and deploy GenAI solutions. According to the company, GALE simplifies GenAI development and purportedly cuts the time by up to 50%, offering a wide-range of use cases that can deliver business value to accelerate adoption. Hey Siri or Alexa, why do we always seem to use female names for GenAI platforms?
Anyway, the aim with GALE is to create a collection of essential AI tools for the enterprise. A complete AI productivity suite encompasses various aspects, but currently in the market, these often lack the depth of specialized products targeting specific market areas. GALE captures most all of these elements in a single no-code (my favorite for adoption) AI productivity suite.
Could this mean faster innovation and quicker responses to market changes? Hopefully. Business leaders know it’s not just about hopping on the new tech bandwagon; it’s about giving every team the tools to grow, innovate, and meet customer demands. Again, what I find important here is not to know how it’s working, but how tools like these can help CXO leaders make an impact to their business—preferably impacts to customer value.
Winning In The Game Of Enterprise Fit
I connected with Vaibhav Bansal, VP of global research firm Everest Group, who was quoted in the release, about what it takes to win in this hyper-competitive, high-stakes game: “As enterprise adoption of GenAI picks up pace, organizations are looking to achieve faster time to value from AI initiatives. They need platforms that offer an easy way to experiment, build, deploy, and scale AI agents and applications. Typical platform features include an AI/ML orchestration layer for efficient model management, the ability to seamlessly connect with enterprise data, a low-code interface, and an application development layer for deploying AI agents or apps.
Beyond these, enterprises need access to the best-performing foundation models, provisions for bringing their own models, guardrails to ensure fairness and data security, the ability to fine-tune models on enterprise data, and pre-configured GenAI libraries and frameworks for different use cases. Enterprise-grade GenAI platforms that support such features promise an exciting future and have the potential to be the one-stop suite for all enterprise AI needs.”
Will GALE shake up the game? Time will tell, but one competitive differentiator seems to be the freedom of choice it brings to the table for models, deployment options, data or applications. Another is the ability to experiment, tune, integrate and deploy at enterprise scale much more quickly than other methods. Since CXOs need ready-to-go models that they can play with to fit their business needs, it plays nice with any LLM you throw at it—commercial, open-source, or custom—giving the oh-so-desired flexibility to adapt as things evolve.
The bottom line is that enterprise CXOs have been waiting for platforms like these—an AI starting point that simplifies the complexities of GenAI building, accelerates adoption, and enhances business processes for long-term value. It is an exciting time to be a CXO enterprise leader—let the next-level games begin.